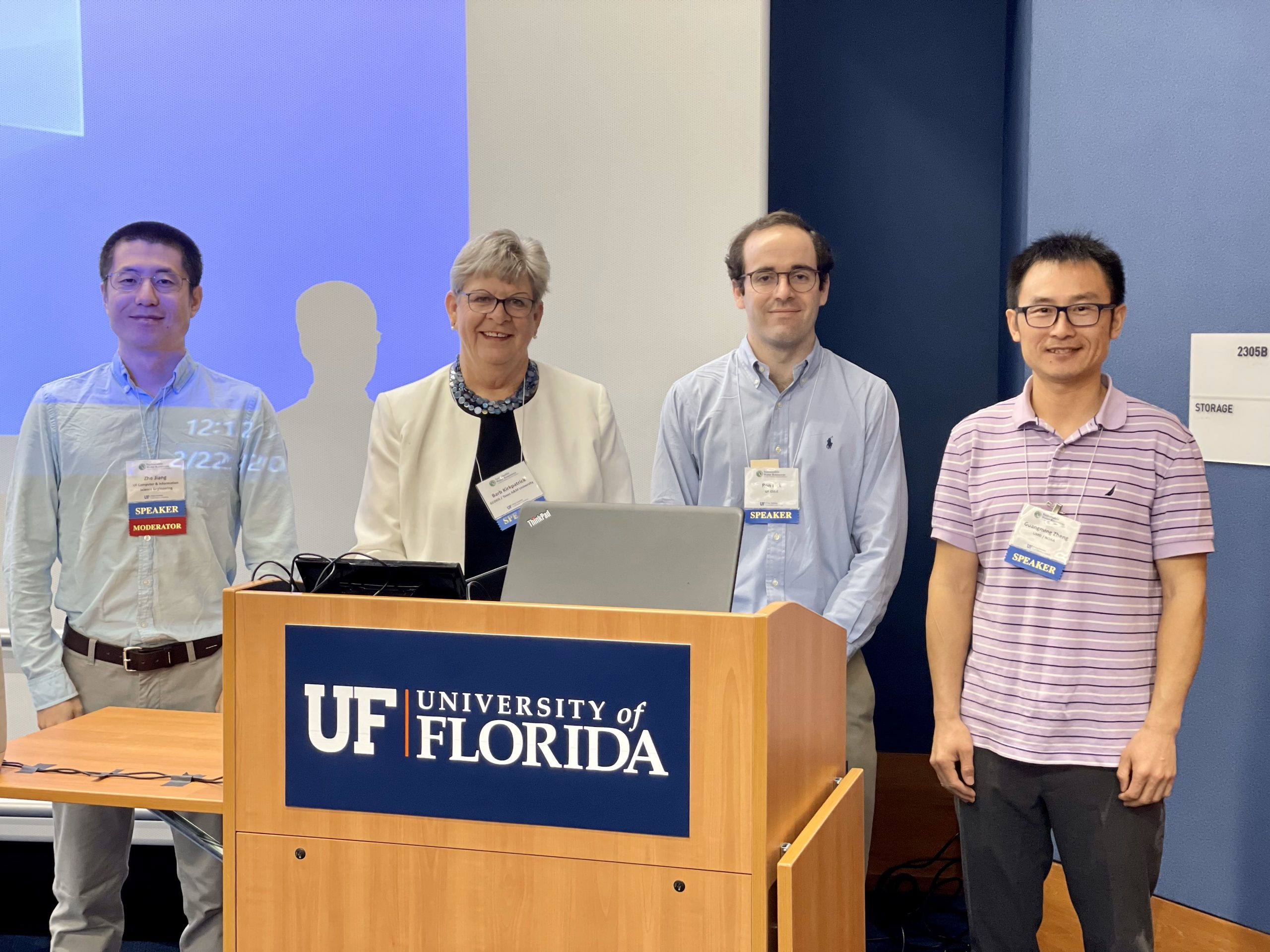
CCS Associate Research Scientist Ron Fick, Ph.D., is harnessing artificial intelligence (AI) to forecast the water quality parameters of red tide blooms and chlorophyll-a concentration (i.e., the amount of algae growing in a body of water) in the Peace River Basin. Fick partners with CCS affiliates Zhe Jiang, Ph.D., and Guangming Zheng, Ph.D., a research associate at NOAA, to analyze satellite and ground data with machine learning methods, to create predictive maps of red tide blooms and chlorophyll-a concentrations.
“The value of AI is that it potentially identifies patterns people can’t see very well,” said Fick.
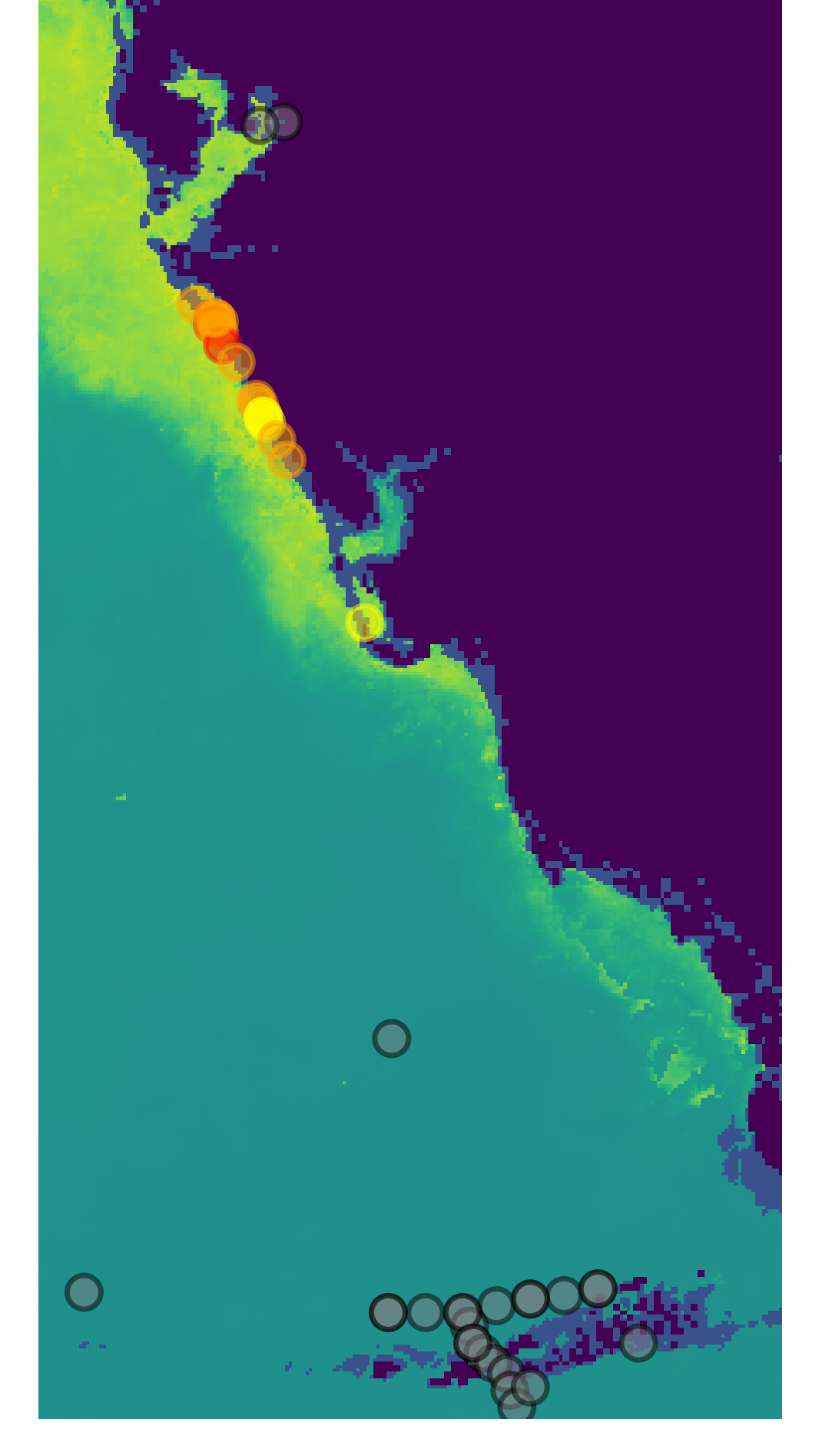
A key advantage of AI is that researchers can feed multiple samples on the ground and satellite data into a model that can oftentimes make predictions better than traditional methods, which are experimental and rely on limited amount of data from the ground.
Machine learning methods can support researchers in predicting concentrations of red tide and chlorophyll-a for mitigation and avoidance strategies. The practical applications range from advising people on which beaches are safe to visit during red tide events, to large-scale implications for economic and health outcomes for people and wildlife.
AI can also be used in conjunction with methods for tackling red tide such as clay mitigation, which involves dumping modified clay particles that bind with red tide organisms and carries them to the seafloor. This method has been used in some local settings and if it were to be scaled up, AI could inform researchers where to target this method.
The next step for this project is to leverage AI to use level one data to predict chlorophyll-a, which is a measure of the amount of algae growing in a body of water. Level one satellite data, the raw data that comes directly from the satellite’s sensors, often needs to be preprocessed using physical models to be ready for analysis. Traditional chlorophyll-a prediction uses level two data, which has been processed and includes higher-level information like geolocation. Fick and his team hypothesize that by using the raw data directly, AI can learn to model the entire process better than how people have been doing it traditionally.
By leveraging AI to draw intelligence from multiple databases, scientists will be able to better predict where and when these toxic algae will bloom as they become increasingly unpredictable and frequent due to climate change and population growth.
This work is part of the Peace River Basin project, a partnership between CCS and SAS to study the factors that influence water quality, and the connections between water quality and economic activity in Charlotte Harbor and the Peace River basin.